Introduction
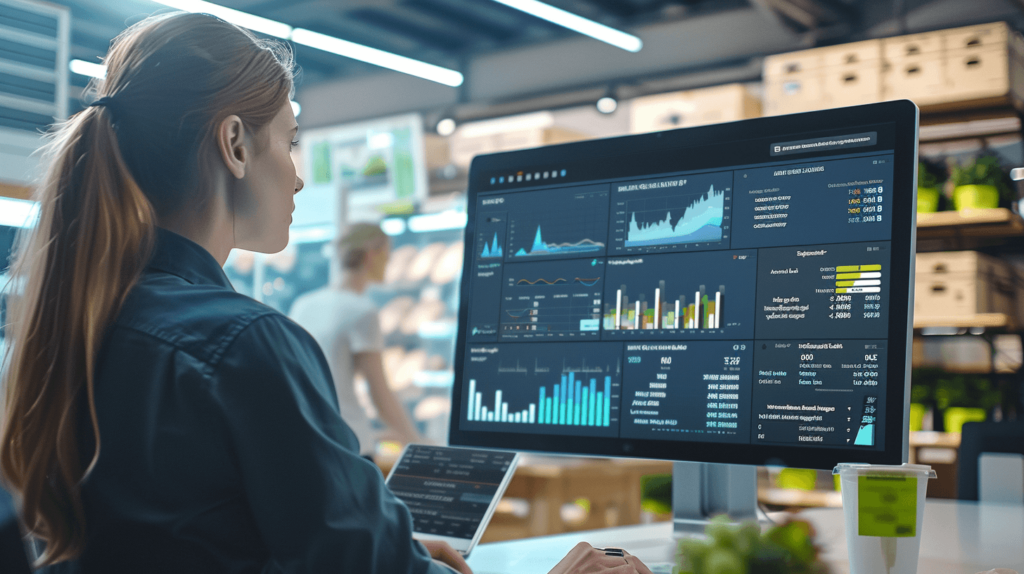
E-commerce is no longer just about offering products online—it’s about crafting personalized experiences that captivate customers. In an industry where competition is fierce, data analytics has become the backbone of successful strategies. However, generic analytics approaches often fall short in addressing unique business challenges.
Enter personalized data analytics support for e-commerce, a solution that turns raw data into actionable insights tailored to specific business needs. Whether it’s improving customer retention, optimizing marketing spend, or boosting conversion rates, personalized analytics ensures your strategies are as unique as your audience.
This article dives into how personalized data analytics can revolutionize e-commerce, offering benefits that go beyond standard metrics and empowering businesses to thrive in a data-driven world.
The Role of Data Analytics in E-commerce
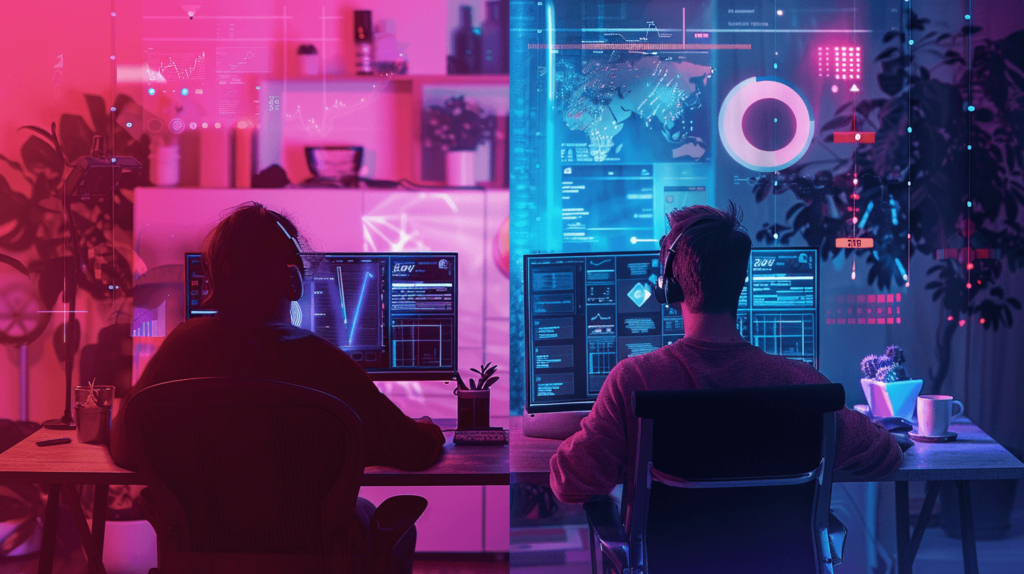
Data analytics has transformed e-commerce from guesswork to precision. Businesses can now understand their customers’ behaviors, preferences, and pain points with unmatched accuracy. But why is this crucial?
Customer Behavior Insights
Imagine knowing exactly when a customer is likely to abandon their cart or what product they’re most inclined to purchase next. Data analytics offers this clarity, enabling businesses to tailor their strategies accordingly.
Marketing Optimization
With data at the core, marketing campaigns become laser-focused. From tracking the ROI of paid ads to determining which email subject lines resonate, analytics empowers businesses to make informed decisions.
Enhanced Decision-Making
Data-driven decision-making reduces risks. By analyzing trends and patterns, e-commerce brands can predict future demands, stock accordingly, and stay ahead of competitors.
For instance, a study by McKinsey highlights that data-driven organizations are 23 times more likely to acquire customers and 19 times more likely to be profitable. Yet, as effective as traditional analytics is, personalization takes it to the next level.
What is Personalized Data Analytics Support?
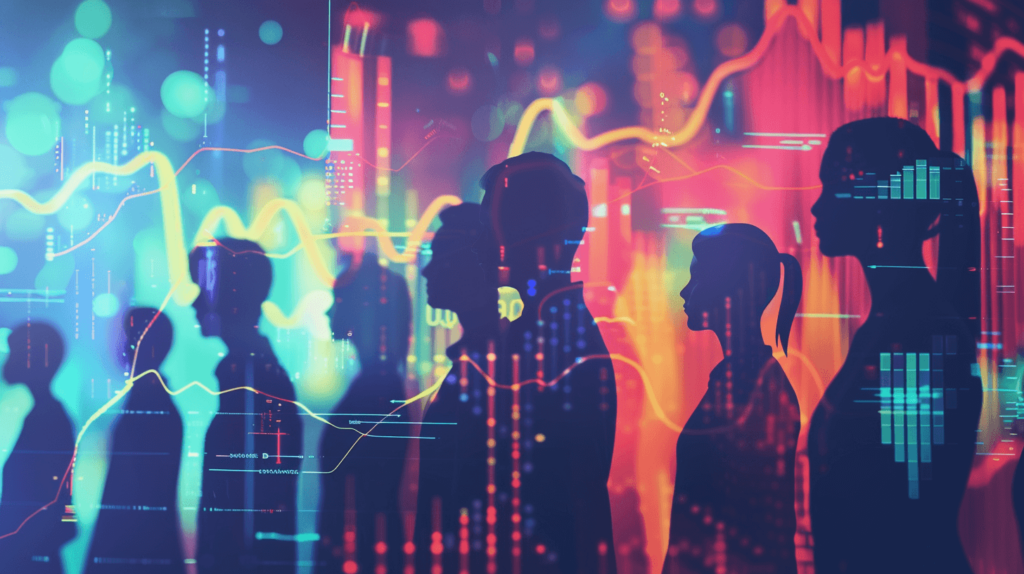
Personalized data analytics support isn’t just about collecting data—it’s about interpreting it in a way that aligns with your e-commerce goals. Unlike one-size-fits-all analytics, this approach focuses on individual business needs, ensuring every insight is actionable and relevant.
Key Elements of Personalized Analytics:
Customer Segmentation
Personalized analytics categorizes customers based on behavior, purchase history, and preferences. This enables hyper-targeted marketing campaigns.
Example: A beauty brand segments customers into those who purchase skincare and those who favor makeup. Tailored email campaigns then promote products within those categories, increasing conversion rates.
Predictive Analytics
Harnessing machine learning, personalized analytics predicts future trends, from peak shopping times to likely product returns.
Real-Time Insights
Traditional analytics might tell you what happened last quarter; personalized analytics shows you what’s happening now, enabling instant action.
Analogy: Think of standard analytics as a map, while personalized analytics is your GPS, guiding you with live updates to ensure you reach your goals efficiently.
Benefits of Personalized Data Analytics Support for E-commerce
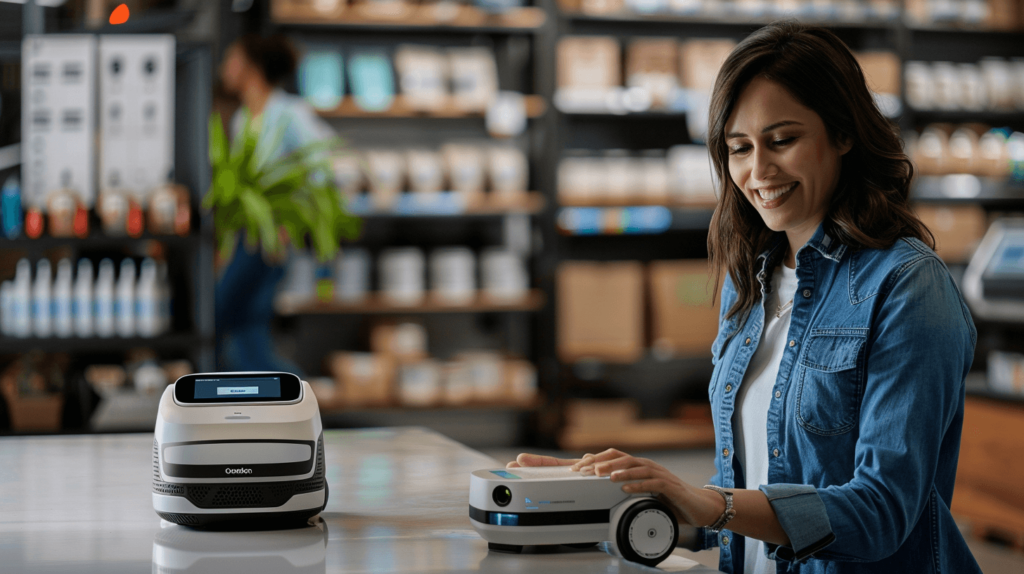
Personalized analytics offers a suite of benefits that can elevate any e-commerce operation. Here’s how:
Enhanced Customer Experience
In e-commerce, personalization equals satisfaction. Personalized recommendations, dynamic pricing, and tailored communications make customers feel valued.
Example: Amazon’s “You May Also Like” feature drives significant sales by showing products based on browsing and purchase history.
Optimized Marketing Campaigns
Personalized data analytics reveals which campaigns resonate with different audience segments. This ensures your ad spend goes further by targeting the right people with the right message.
Pro Tip: Use analytics to track click-through rates (CTR) and conversion rates across different demographics, then fine-tune your campaigns.
Better Inventory Management
Nothing frustrates customers like out-of-stock products. Personalized analytics predicts demand trends, helping you maintain optimal inventory levels.
Real-Life Insight: A fashion retailer used predictive analytics to identify which items would trend during summer, ensuring they had adequate stock to meet demand.
Increased ROI
Businesses that use personalized analytics allocate resources more effectively, avoiding wasteful spending and focusing on high-impact strategies.
Stat Highlight: A recent study found that personalization can boost marketing ROI by 30%.
Actionable Insights for Growth
Personalized analytics doesn’t just show numbers—it highlights opportunities. Whether it’s a new product line or an untapped customer segment, these insights drive growth.
Example: A small e-commerce store specializing in eco-friendly goods identified a growing interest in reusable water bottles through analytics. By introducing this product, they saw a 25% revenue increase in six months.
Implementing Data Analytics in Your E-Commerce Business
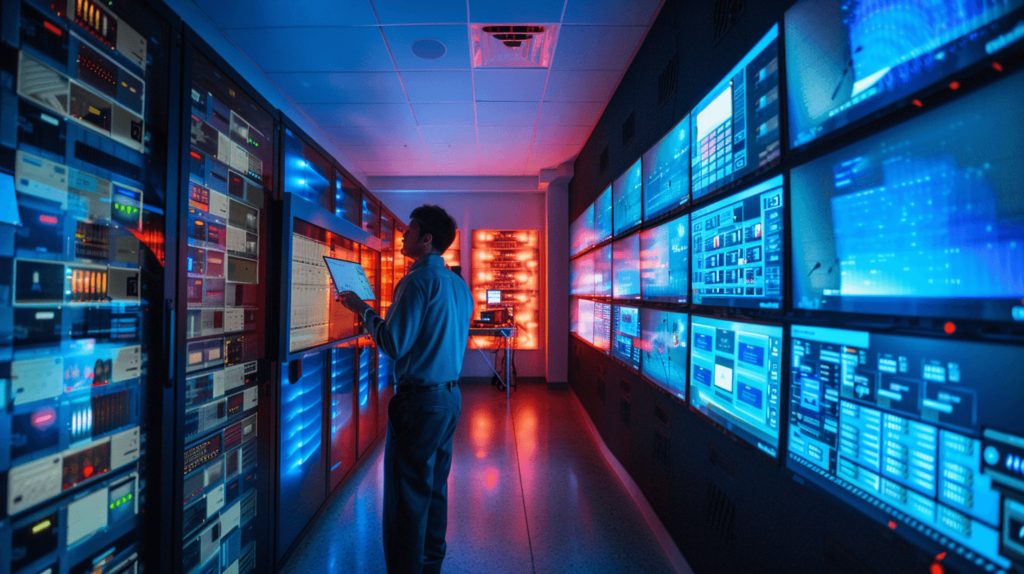
The journey to harnessing the full potential of personalized data analytics support for e-commerce begins with a strategic and thoughtful implementation. This involves integrating analytics tools, setting measurable goals, and cultivating a data-driven culture within your organization.
Building the Infrastructure for Data Analytics
Effective data analytics requires a robust infrastructure. Start by investing in reliable software and systems that align with your business needs.
Key Components of a Strong Analytics Framework:
Data Collection Tools: Leverage platforms like Google Analytics, Shopify Analytics, or specialized e-commerce data tools to capture key metrics.
Integration with E-Commerce Platforms: Ensure seamless integration with platforms like WooCommerce, Magento, or BigCommerce to centralize data.
Cloud Solutions: Use cloud-based analytics platforms for scalability, enhanced storage, and real-time access to data.
Example: A mid-sized e-commerce retailer implemented Shopify Analytics alongside Looker for advanced data visualization. This streamlined their reporting process, giving the team a comprehensive view of customer behavior.
Personalizing Analytics for Deeper Insights
Personalization in data analytics isn’t just about creating dashboards; it’s about uncovering insights that directly impact your unique business model.
How to Personalize Your Analytics:
Segment Customer Data: Break down your audience by demographics, purchase history, and preferences. This helps identify specific trends and opportunities.
Track Tailored KPIs: Identify and monitor metrics that align with your goals, such as average order value (AOV), conversion rates, or customer acquisition costs.
Create Custom Reports: Build reports that focus on your niche, whether it’s seasonal trends, high-performing products, or abandoned cart recovery.
Pro Tip: Automate personalized reports for key stakeholders to ensure decision-makers have access to timely, relevant data.
Leveraging Predictive Analytics
Predictive analytics transforms raw data into foresight, helping e-commerce businesses anticipate trends and customer needs. By analyzing historical data, predictive tools forecast future behaviors, enabling proactive strategies.
Applications of Predictive Analytics:
Inventory Management: Forecast demand for products to prevent overstocking or stockouts.
Customer Retention: Use predictive modeling to identify at-risk customers and create targeted retention campaigns.
Pricing Strategies: Analyze market trends to optimize pricing dynamically for maximum profitability.
Example: A subscription box company used predictive analytics to anticipate customer churn. By identifying high-risk customers, they implemented personalized discounts and offers, reducing churn by 20%.
Advanced Data Analytics Techniques for E-Commerce
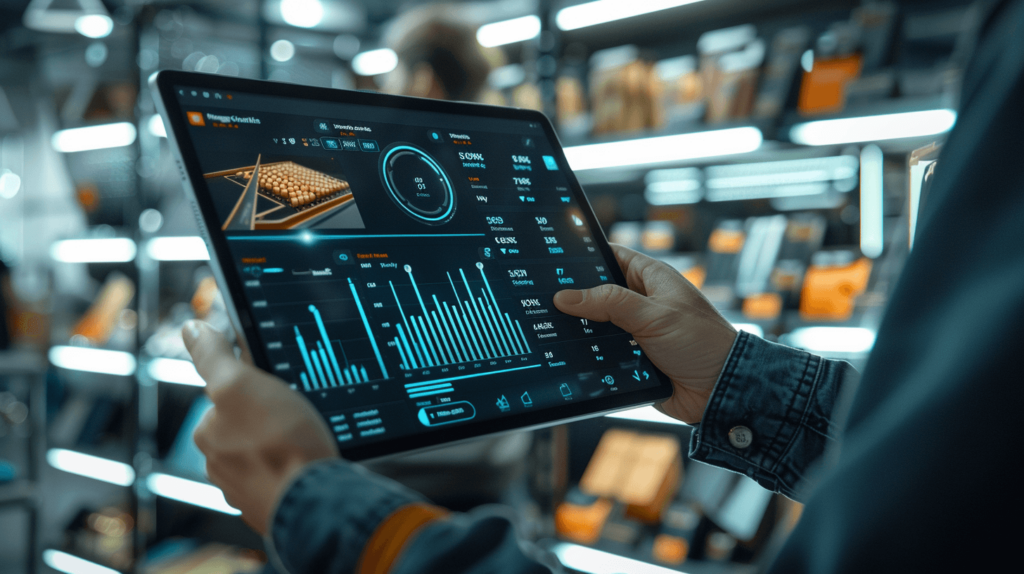
Once the foundation is established, advanced analytics techniques can help elevate your business to the next level.
A/B Testing and Experimentation
Experimentation is essential for optimizing your e-commerce strategy. A/B testing involves comparing two versions of a webpage, email, or product page to see which performs better.
Steps for Effective A/B Testing:
Set a Clear Hypothesis: Define what you want to test (e.g., button color, headline text, product placement).
Analyze Results: Use analytics tools to measure performance differences in metrics like click-through rates, sales, or engagement.
Implement Winning Variants: Apply the most successful elements to improve your overall strategy.
Case Study: An online apparel retailer tested different product page layouts. By highlighting customer reviews more prominently, they increased conversions by 15%.
Machine Learning and AI Integration
Artificial intelligence (AI) and machine learning are revolutionizing e-commerce analytics by automating processes and uncovering deep insights.
Key Benefits of AI in Analytics:
Personalized Recommendations: Use AI algorithms to suggest products based on customer preferences and behavior.
Dynamic Pricing: Adjust prices in real-time based on demand, competitor pricing, and customer segmentation.
Fraud Detection: Identify unusual transactions or patterns that may indicate fraudulent activity.
Example: A global marketplace integrated AI-driven analytics to refine its product recommendation engine. As a result, they saw a 25% increase in average order value.
Real-Time Analytics for Immediate Action
Real-time analytics enables e-commerce businesses to make data-driven decisions instantly. By monitoring live metrics, you can respond to customer behaviors as they happen.
Real-Time Use Cases:
Flash Sales: Adjust inventory or promote high-demand items during flash sales.
Customer Support: Identify and resolve bottlenecks in the checkout process immediately.
Social Media Monitoring: Track and capitalize on trending hashtags or viral products.
Pro Tip: Use tools like Tableau or Power BI to visualize real-time data for actionable insights.
Measuring the ROI of Personalized Data Analytics
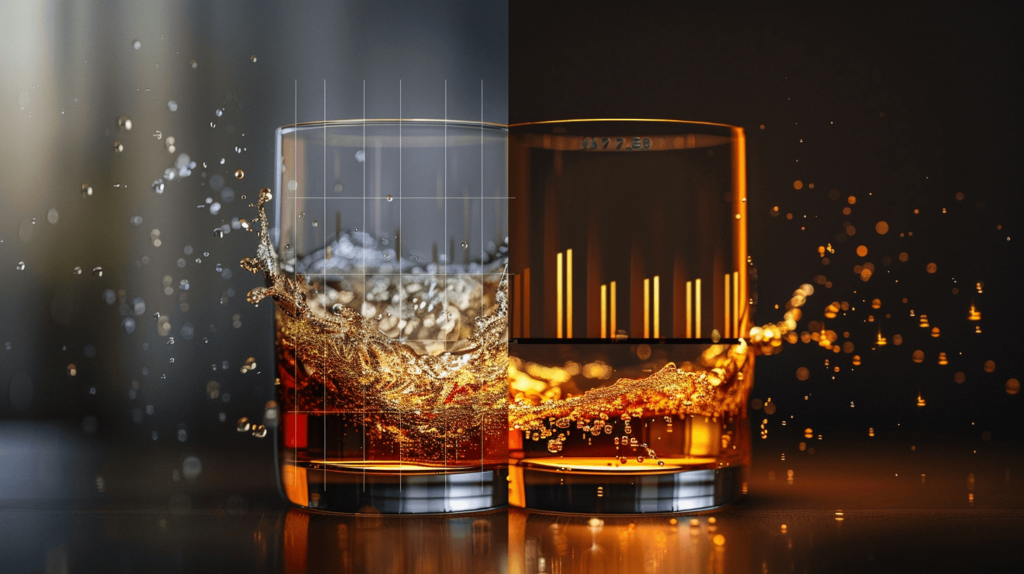
To ensure your data analytics strategy is effective, measuring its return on investment (ROI) is crucial.
How to Calculate ROI:
Set Baselines: Compare key metrics like sales and conversion rates before and after implementing analytics solutions.
Monitor Improvements: Track increases in revenue, customer satisfaction, and operational efficiency.
Analyze Cost Savings: Factor in reductions in marketing spend, inventory waste, and customer acquisition costs.
Example: A beauty brand invested in personalized analytics to improve email targeting. By tailoring campaigns to customer preferences, they achieved a 40% increase in open rates and a 25% boost in sales, far outweighing their initial investment.
Conclusion: Unlock the Power of Data-Driven Growth
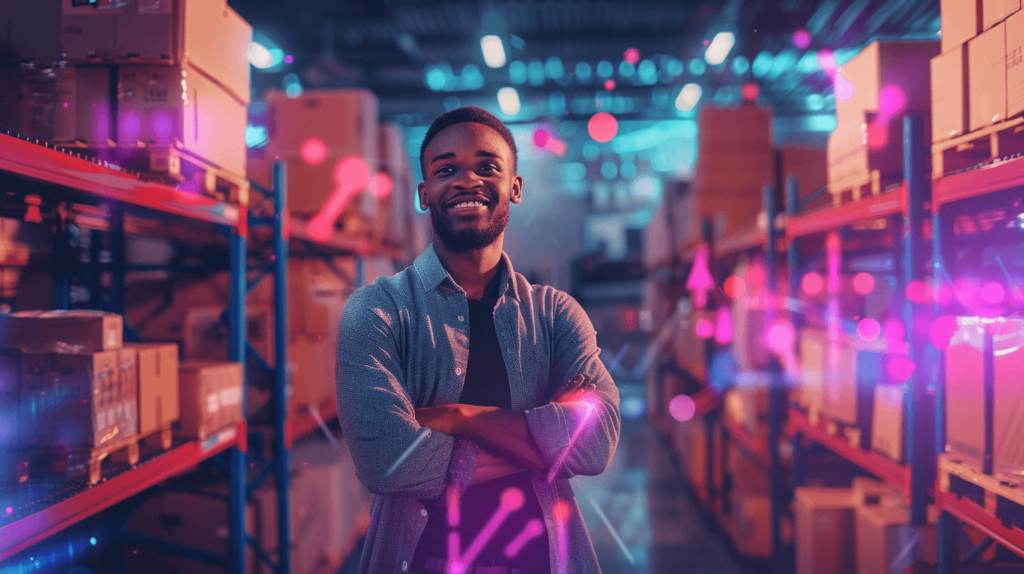
Personalized data analytics support for e-commerce is no longer optional—it’s essential for thriving in today’s competitive market. By leveraging tailored insights, predictive modeling, and advanced analytics techniques, businesses can optimize operations, engage customers, and drive sustained growth.
Whether you’re an emerging brand or a seasoned retailer, embracing analytics empowers you to stay ahead of trends, respond to customer needs, and create memorable shopping experiences.
Ready to transform your e-commerce business with data analytics? Explore our tailored solutions to unlock actionable insights and elevate your strategy. Explore our website to learn more and start your journey toward data-driven success. Let’s grow together!